How Predictive Maintenance Works
Predictive maintenance utilizes advanced data analytics to predict when equipment failures might occur before they actually do. By collecting and analyzing real-time data from sensors and other sources, maintenance teams can identify patterns and trends indicative of potential issues. This proactive approach allows them to schedule maintenance tasks efficiently, minimizing downtime and reducing overall maintenance costs.
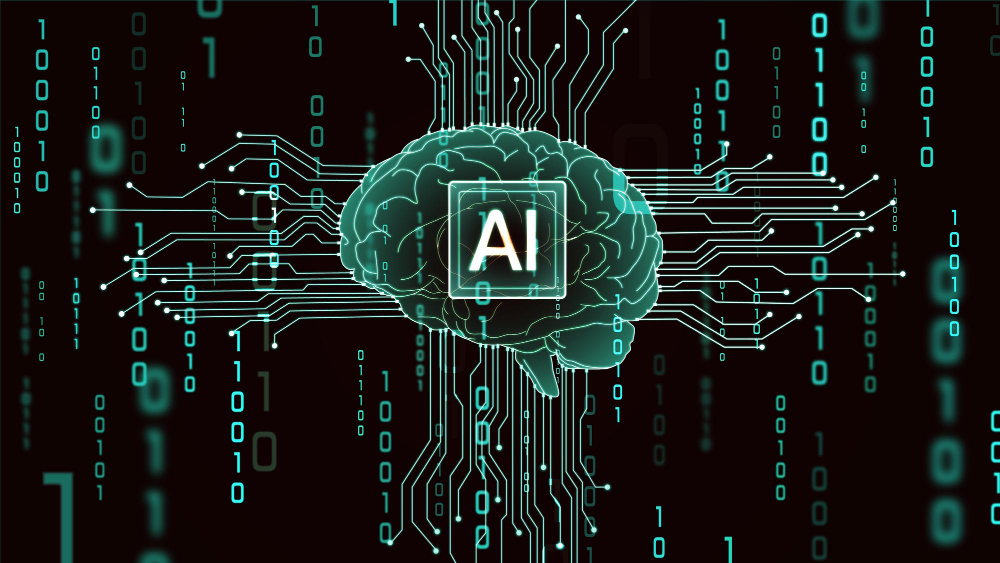
Through the use of predictive modeling and machine learning algorithms, predictive maintenance can forecast equipment failures based on historical data and current operating conditions. By monitoring key performance indicators and running predictive analytics, maintenance teams can make data-driven decisions to optimize maintenance schedules and ensure equipment reliability. This data-driven approach shifts maintenance from a reactive to a proactive strategy, enabling organizations to better manage their assets and improve operational efficiency.
Benefits of Implementing Predictive Maintenance
Predictive maintenance offers numerous benefits to organizations across various industries. One significant advantage is the ability to prevent unexpected breakdowns and equipment failures by identifying potential issues before they escalate. This proactive approach helps minimize downtime, increase equipment reliability, and ultimately reduce maintenance costs. Additionally, predictive maintenance enables companies to schedule maintenance during planned downtimes, optimizing operational efficiency and ensuring continuous production without disruptions.
Another key benefit of implementing predictive maintenance is the enhancement of overall equipment effectiveness (OEE). By monitoring equipment health in real-time and predicting maintenance needs, organizations can improve asset performance and maximize productivity. This leads to increased equipment lifespan, improved product quality, and enhanced safety for workers. Ultimately, predictive maintenance empowers businesses to make data-driven decisions, streamline maintenance processes, and achieve higher operational efficiency.
Challenges Faced in Predictive Maintenance
One common challenge faced in predictive maintenance is the issue of data quality and quantity. For accurate predictions to be made, a large amount of high-quality data is required. However, obtaining this data can be a cumbersome task, especially if the necessary sensors and monitoring systems are not in place. Inaccurate or insufficient data can lead to flawed predictions and undermine the effectiveness of the maintenance program.
Another challenge is the integration of predictive maintenance systems with existing infrastructure and processes. Many organizations struggle with incorporating new technologies into their established workflows, which can result in resistance to change and slow adoption rates. Ensuring seamless integration and alignment with existing systems is crucial for the successful implementation of predictive maintenance practices. This requires careful planning, stakeholder buy-in, and effective communication throughout the organization.
Different Types of Predictive Maintenance Techniques
Predictive maintenance techniques can be broadly categorized into four main types: vibration analysis, oil analysis, infrared thermography, and ultrasonic testing. Vibration analysis involves monitoring the vibration levels of machinery to detect any anomalies that could indicate potential issues. Oil analysis focuses on examining the properties of lubricants to identify wear particles or contaminants that may indicate equipment degradation. Infrared thermography uses thermal imaging to identify overheating components in machinery, which can point towards impending failures. Ultrasonic testing detects changes in sound frequencies emitted by equipment to detect faults such as leaks or mechanical issues before they escalate. Each of these techniques plays a crucial role in enabling proactive maintenance practices, thereby reducing the likelihood of unexpected breakdowns and increasing overall operational efficiency.
The Evolution of Predictive Maintenance with Artificial Intelligence
Predictive maintenance has witnessed a significant evolution with the integration of artificial intelligence (AI) technologies. Traditionally, maintenance schedules were often based on fixed intervals or reactive responses to machine failures. However, AI has revolutionized this approach by enabling predictive maintenance strategies that rely on data-driven insights and algorithms to predict equipment failures before they occur.
AI-powered predictive maintenance solutions utilize advanced analytics, machine learning algorithms, and real-time data monitoring to forecast potential issues and optimize maintenance processes. By analyzing historical data patterns and equipment performance metrics, AI can identify subtle anomalies indicating potential faults, allowing maintenance teams to proactively address maintenance needs. This shift towards predictive maintenance with AI has resulted in improved operational efficiency, reduced downtime, and cost savings for organizations across various industries.
• AI technologies have enabled predictive maintenance strategies based on data-driven insights
• Predictive maintenance solutions utilize advanced analytics, machine learning algorithms, and real-time data monitoring
• AI can identify subtle anomalies indicating potential faults to proactively address maintenance needs
• The shift towards predictive maintenance with AI has resulted in improved operational efficiency, reduced downtime, and cost savings for organizations across various industries
Role of Machine Learning in Predictive Maintenance
Machine learning plays a pivotal role in predictive maintenance by enabling the automation of data analysis to identify patterns that indicate potential equipment failures. This technology utilizes algorithms to continuously learn from new data and improve its predictive capabilities over time. By analyzing historical performance data and real-time sensor information, machine learning algorithms can forecast when maintenance is required, allowing for timely interventions to prevent costly downtime.
Furthermore, machine learning algorithms can prioritize maintenance tasks based on the severity of potential issues, optimizing resource allocation and maximizing equipment uptime. This predictive approach helps companies shift from reactive maintenance practices to proactive strategies, saving both time and money in the long run. Overall, the integration of machine learning in predictive maintenance empowers organizations to make data-driven decisions that enhance operational efficiency and prolong the lifespan of their assets.
Impact of IoT in Predictive Maintenance
Predictive maintenance has seen a significant transformation with the integration of IoT (Internet of Things) technologies. IoT enables the continuous monitoring of equipment and machinery in real-time, providing valuable data on their performance and health. By collecting data from sensors and devices embedded in machines, organizations can detect potential issues early on, allowing for timely maintenance before breakdowns occur. This proactive approach minimizes downtime, reduces maintenance costs, and extends the lifespan of assets.
Furthermore, IoT facilitates remote monitoring and diagnosis, enabling maintenance teams to access equipment data from anywhere at any time. This capability enhances the efficiency of maintenance operations and empowers organizations to implement predictive maintenance strategies more effectively. With IoT, organizations can transition from reactive or preventive maintenance approaches to predictive maintenance, optimizing their maintenance schedules and improving overall operational efficiency.
Integration of Data Analytics in Predictive Maintenance
Data analytics plays a crucial role in enhancing the effectiveness of predictive maintenance strategies. By integrating data analytics, organizations can harness the power of historical data, real-time information, and predictive models to anticipate potential equipment failures. This proactive approach enables maintenance teams to schedule timely interventions, reducing downtime and optimizing equipment performance.
Furthermore, the integration of data analytics in predictive maintenance allows for the implementation of condition-based monitoring systems. By continuously monitoring equipment health indicators, organizations can detect anomalies and trends early on, leading to faster decision-making processes and improved maintenance planning. Ultimately, leveraging data analytics in predictive maintenance not only enhances operational efficiency but also contributes to cost savings and increased productivity.
Advantages of Using AI in Predictive Maintenance
AI offers numerous advantages in predictive maintenance that can significantly improve the efficiency and effectiveness of maintenance processes. One key advantage is the ability of AI algorithms to analyze large volumes of data quickly and accurately, providing insights into equipment performance and potential failures in real-time. This enables maintenance teams to proactively address issues before they escalate, minimizing downtime and reducing overall maintenance costs.
Additionally, AI can help in optimizing maintenance schedules by predicting the remaining useful life of equipment components based on historical data and current operating conditions. This predictive capability allows organizations to schedule maintenance tasks more efficiently, reducing unnecessary maintenance activities and ensuring that resources are allocated where they are most needed. Overall, the use of AI in predictive maintenance can lead to increased reliability, improved asset performance, and enhanced operational efficiency.
Case Studies of Successful Predictive Maintenance Implementations
One notable case study showcasing successful implementation of predictive maintenance is that of a large manufacturing company that utilized advanced AI algorithms to monitor the performance of their machinery in real-time. By analyzing sensor data, the company was able to predict potential equipment failures before they occurred, allowing for timely maintenance interventions and minimizing costly downtime.
Another inspiring example comes from the aviation industry, where a major airline implemented predictive maintenance techniques to optimize the performance of their aircraft fleet. Through the use of IoT sensors and data analytics, the airline was able to accurately predict component failures and schedule maintenance activities proactively. This approach not only improved the overall reliability of their aircraft but also resulted in significant cost savings by reducing unexpected maintenance expenses.
Future Trends in Predictive Maintenance Technologies
As technology continues to advance, the future of predictive maintenance technologies is poised for significant growth and innovation. One key trend that is expected to shape the landscape is the increased integration of machine learning algorithms into predictive maintenance systems. By leveraging complex algorithms and historical data, machine learning can help anticipate potential equipment failures with greater accuracy, enabling proactive maintenance interventions to be scheduled promptly.
Another trend that is gaining momentum in the realm of predictive maintenance technologies is the utilization of sensor data fusion. By combining data from multiple sensors installed on equipment, maintenance professionals can obtain a more comprehensive understanding of asset health and performance. This holistic approach allows for more informed decision-making and can lead to increased operational efficiency and reduced downtime.
Best Practices for Implementing AI in Predictive Maintenance
One of the key best practices for successful implementation of AI in predictive maintenance is to ensure a high-quality data infrastructure. This involves collecting relevant data from various sources, cleaning and preprocessing it effectively, and storing it securely in a centralized database. Having a solid data foundation is crucial for training AI algorithms and extracting valuable insights for predictive maintenance tasks.
Another important best practice is to continuously evaluate and fine-tune the AI models used in predictive maintenance. This requires monitoring the performance of the models regularly, identifying any discrepancies or anomalies, and updating the algorithms as needed to maintain their accuracy and efficiency. By iteratively refining the AI models based on real-world feedback and data, organizations can enhance the predictive capabilities of their maintenance strategies and optimize equipment reliability and performance.
Conclusion and Recommendations
In conclusion, predictive maintenance, coupled with advanced technologies such as artificial intelligence and machine learning, offers a proactive approach towards ensuring the smooth operation of machinery and equipment. By harnessing the power of data analytics and IoT, organizations can optimize their maintenance processes, reduce downtime, and ultimately enhance operational efficiency.
As organizations continue to embrace predictive maintenance techniques, it is crucial for them to focus on building a robust data infrastructure, investing in employee training, and staying updated with the latest technological developments in the field. By adhering to best practices and continuously refining their predictive maintenance strategies, businesses can unlock significant cost savings, improve asset reliability, and gain a competitive edge in today’s fast-paced industrial landscape.
How does predictive maintenance work?
Predictive maintenance involves using data and analytics to predict when equipment is likely to fail, allowing for proactive maintenance to be performed before a breakdown occurs.
What are the benefits of implementing predictive maintenance?
Some benefits of implementing predictive maintenance include reduced downtime, lower maintenance costs, increased equipment lifespan, and improved operational efficiency.
What are some challenges faced in predictive maintenance?
Challenges in predictive maintenance can include data quality issues, lack of skilled personnel, integration with existing systems, and upfront costs of implementing new technologies.
What are the different types of predictive maintenance techniques?
Some common types of predictive maintenance techniques include vibration analysis, infrared thermography, oil analysis, ultrasonic testing, and motor current analysis.
How has predictive maintenance evolved with artificial intelligence?
Artificial intelligence has enabled predictive maintenance to become more advanced and accurate, allowing for more precise predictions of when maintenance is needed and better optimization of maintenance schedules.
What is the role of machine learning in predictive maintenance?
Machine learning algorithms can analyze large amounts of data to identify patterns and anomalies that can be used to predict equipment failures and optimize maintenance schedules.
How does IoT impact predictive maintenance?
The Internet of Things (IoT) enables real-time monitoring of equipment and assets, providing valuable data for predictive maintenance analysis and allowing for remote diagnostics and maintenance.
How is data analytics integrated into predictive maintenance?
Data analytics is used to process and analyze large amounts of data collected from equipment sensors and other sources to make predictions about when maintenance is needed and identify trends and patterns.
What are the advantages of using AI in predictive maintenance?
Using AI in predictive maintenance can lead to more accurate predictions, reduced downtime, lower maintenance costs, improved equipment reliability, and better overall operational efficiency.
Can you provide some case studies of successful predictive maintenance implementations?
Yes, case studies of successful predictive maintenance implementations can be found in various industries, showcasing the benefits of using advanced technologies for maintenance optimization.
What are some future trends in predictive maintenance technologies?
Future trends in predictive maintenance technologies include the use of more advanced AI algorithms, increased integration with IoT devices, and the adoption of predictive analytics for more predictive accuracy.
What are some best practices for implementing AI in predictive maintenance?
Best practices for implementing AI in predictive maintenance include defining clear objectives, ensuring data quality, training personnel on new technologies, and continuously monitoring and evaluating performance for improvement.
In conclusion, what are the key recommendations for companies looking to implement predictive maintenance?
Companies looking to implement predictive maintenance should focus on investing in advanced technologies, training personnel on new tools, ensuring data quality and accuracy, and continuously evaluating and optimizing maintenance strategies for better efficiency and cost-effectiveness.